Revolutionizing Clinical Trials: How AI Streamlines Design and Reduces Failure Rates
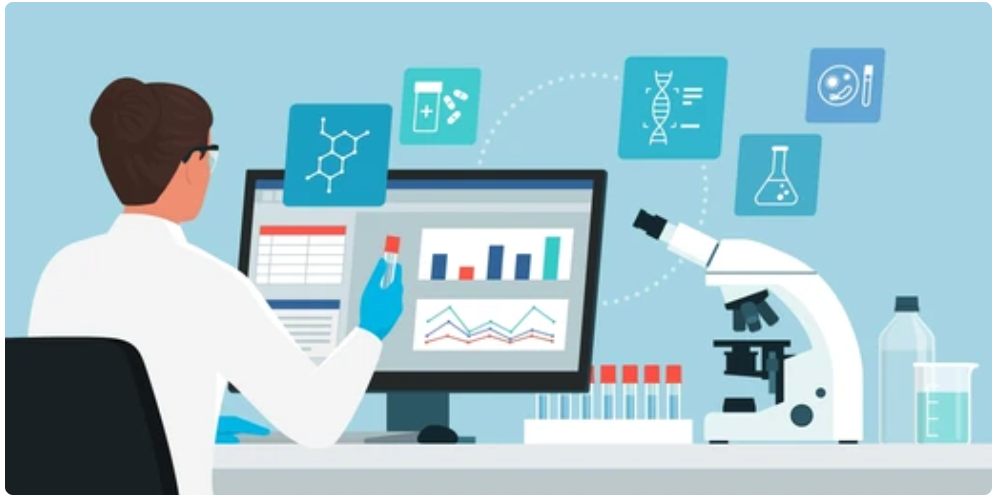
To bring a new drug to the market takes approximately 10-15 years and costs between 1.5-2 billion US dollars. As such, a failed trial can result in significant costs in terms of both time and money. Unfortunately, clinical trial failure is not a rare occurrence; only 1 in 10 compounds that enter trials reach the market. Fundamental causes of trial failure include poor patient cohort selection, recruitment, and retainment, poor patient monitoring, and safety issues. The development of medicine relies on successful clinical trials which are indispensable in bringing innovative therapeutics from the laboratory to patients. Nevertheless, as our understanding of human complexity grows, clinical trial design is becoming increasingly challenging.
Fortunately, AI has proven incredibly useful in transforming critical steps of clinical trial design, helping to overcome some of the issues that ultimately result in clinical trial failure. Innovative AI platforms have proven useful, safe, and cost-effective in clinical trial optimization. NextTrial AI offers a highly collaborative, AI-driven, cloud-based data platform that simplifies all stages of the clinical trial lifecycle from inception to commercialization. It provides a unified data repository with AI-powered decision support and complete trial oversight that streamlines the trial process, ultimately minimizing the risk of failure and expediting research translation.
NextTrial AI in Clinical Trial Design
NextTrial AI supports clinical trial design at all stages, including selecting optimal endpoints, refining inclusion and exclusion (I/E) criteria, optimizing the assessment schedule, and building a risk profile using safety data. It can also provide support with other required tools for research and analysis.
Endpoint Selection
Selection and refinement of meaningful, clinically relevant endpoints are pivotal for evaluating intervention effects in clinical trials. Endpoint validity should be verified against gold standards, and investigators must estimate the required sample size to achieve study objectives. NextTrial AI’s platform, which combines a unified data repository and AI-powered decision support, streamlines the process of endpoint selection and sample size determination while optimizing endpoint choices to enhance intervention product efficacy. Real-World Data (RWD) and Real-World Evidence (RWE) provide novel insights into the effectiveness of interventions in real-world clinical practice, complementing data collected from clinical trials by reflecting the diversity of patient populations and healthcare settings that may not be represented in traditional clinical trials. Thus, utilizing RWD and RWE in trial design further allows for novel endpoint discovery and valuable insights into rare diseases, supporting a patient-centric approach.
I/E Criteria Refinement
Developing and refining I/E criteria can be a long and complex process with several issues that may effectuate trial failure. Firstly, the design may be too stringent and exclude relevant patients, biasing the results into a favorable outcome. Alternatively, there is a possibility of including a patient population that may lead to a suboptimal outcome or overlooking an underrepresented patient group. In addition to the risk of suboptimal cohort selection, manually sifting through pages of I/E criteria from hundreds of similar trials is a laborious task, worsened by the fact that clinical terms used in I/E criteria are neither standardized nor harmonized.
NextTrial AI proprietary Natural Language Processing (NLP) models are generated from selectively mined features from relevant sources, including past trials and literature. They can successfully yield information on the target characteristics or patient populations that are most likely to produce a favorable clinical outcome for any given endpoint or disease. Additionally, they uncover meaningful clinical entities and hidden relations from unstructured data that would build optimal patient characteristics. This way, I/E criteria variables from many sources can be aggregated and summarized quickly to generate critical insights on several dimensions. Then, extracted patient characteristics can be leveraged using data from disease registries and electronic health records (EHR) to improve recruitment even further.
Schedule of Assessments
During trial design, it is crucial to identify assessment procedures that are necessary for obtaining the required trial data. Nonetheless, investigators should eliminate non-essential procedures to reduce participant burden, enhance patient recruitment, and minimize dropout rates. With NextTrial AI, once the disease profile and study characteristics are optimized, the system can quickly provide a recommended list of procedures from similar trials that will generate the necessary data to support the clinical hypothesis, enhancing participant recruitment and retention. As the trial progresses, the platform provides intelligent insight into participant adherence and behavior, further enhancing participant engagement.
Safety Monitoring
NextTrial AI allows users to harness signals from RWD, historical adverse effect (AE) data, regulatory databases, relevant literature, and approved labels. This supports the development of a favorable benefit-risk profile, crucial for target intervention approval. The ready availability of this data saves users time and effort and provides assurance that all risks have been considered, ensuring patients receive safe and effective treatments, while also minimizing the risk of harm.
Other Relevant Tools for Research and Analysis
In addition to the processes detailed above, NextTrial AI renders it straightforward to harness data and sources required for later use in research and analysis. This could include providing a repository of biomedical literature and past trial data or information on toxicology reports, genes and biomarkers. With NextTrial AI, all relevant information can be sourced, searched, summarized, and seamlessly implemented into the study design. This feature has a two-fold benefit: it not only enhances productivity by saving users time and effort on tedious tasks, but it also facilitates the optimal design of trials with enhanced integrity, reduced human error, and lower risk of unconscious outcome bias.
Summary and Future Perspectives
NextTrial AI utilizes data from past trials, RWD, data available in the literature, and other clinical domains, including in-house data, to simplify the trial design process, helping users to design optimal protocols and reduce protocol amendments. This streamlines the trial design process and minimizes the time to bring innovative treatments to patients. Since its launch last year, NextTrial AI has been continuously enriched with data from diverse sources, including past trials, literature, genomics, AEs, and disease registries, as well as novel predictive models. Over the coming years, with the help of platforms like NextTrial, clinical research processes are set to become more optimized, streamlined, and successful than ever, ultimately reducing the rate of trial failure and improving the global healthcare landscape.